
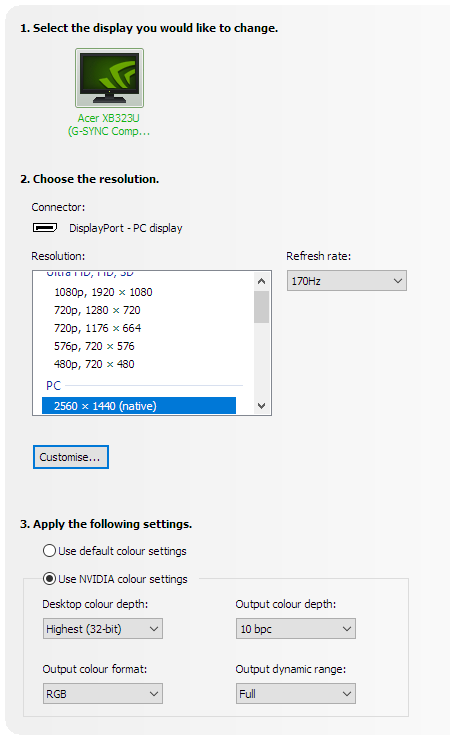
Current methods further combine different tests to identify driver genes, e.g. Typically, these methods aggregate mutation counts over genes or genomic regions, and compare them with a context-dependent background expectation 9, 12, 13. Recent approaches further calibrate their background models to the mutability of different nucleotide contexts 9, 12, 13. To detect the excess of driver mutations over a carefully modeled background, current methods model regional variation in mutation rate with the help of synonymous mutations or epigenomic features 10- 13. For most positions in the genome, functional importance is not known 17, 18 and is usually proxied by differences between synonymous and nonsynonymous mutations 12, positional clustering of mutations 7, and bioinformatically predicted scores of functional significance 8, 9. Applied to thousands of tumor exomes, these methods have contributed greatly to our understanding of which genes are involved in carcinogenesis 5, 11, 12, 14- 16.Ĭurrent algorithms generally exploit two features of driver mutations: first they occur in functionally important genomic positions corresponding to amino acids that are critical for the protein function 6- 9 and second they occur in excess over the background mutability of the genome owing to positive selection in the tumor 10- 13. A number of highly sophisticated computational methods have been developed to identify driver mutations 6- 13. A major goal of cancer genomics is to identify these rare driver mutations amid the myriad passengers 5. Only a small proportion of the somatic mutations found in tumor cells drive tumor development 1- 3, whereas the vast majority are functionally neutral passengers that do not confer selective advantage to cancer cells 4. Our study provides a resource of driver genes across 28 tumor types with additional driver genes identified based on mutations in unusual nucleotide contexts.

We applied our method to whole-exome sequencing data from 11,873 tumor-normal pairs and identified 460 driver genes that clustered into 21 cancer-related pathways. We therefore developed a method that combines this feature with the signals traditionally used for driver gene identification. We observed that mutations in contexts that deviate from the characteristic contexts around passenger mutations provide a signal in favor of driver genes. While passenger mutations are enriched in characteristic nucleotide contexts, driver mutations occur in functional positions, which are not necessarily surrounded by a particular nucleotide context.

Current approaches identify driver genes based on mutational recurrence, or they approximate the functional consequences of nonsynonymous mutations using bioinformatic scores. Cancer genomes contain large numbers of somatic mutations, but few of these mutations drive tumor development.
